Let’s rewind to a time before quarantine and face masks—the year 2019. As we set our sights on 2020, one of our goals at Index was to invest in exchange efficiency on behalf of the media owners and buyers transacting through our exchange. We could never have anticipated how important this goal would become, and realized the time was right to meet 2020’s businesses challenges head-on via machine learning.
Surprise challenges in 2020
With the rise of COVID-19 and the resulting economic downturn, we saw massive increases in traffic as quarantined users spent more time browsing and consuming content, while many programmatic marketers paused their campaigns. At the same time, travel restrictions made it more difficult to install additional hardware in our global data centers. We had already anticipated growing our volume of auctions this year, but these circumstances made it increasingly important that our infrastructure costs did not grow linearly with increased supply.
Case in point—a pretty typical day on the exchange today has us running over 120,000,000,000 auctions (120 billion!), and peaking at over 1.2 million queries per second (QPS).
Media buyers were also challenged by this traffic growth. Many demand-side platforms (DSPs) have QPS constraints that were now being pushed to their limits. To be a good partner, we wanted to deliver solutions that optimized the supply we sent to DSPs, without overwhelming them with supply they weren’t interested in—or simply could not handle given the unexpected surges we were seeing.
Predicting the future with intelligent traffic filtration
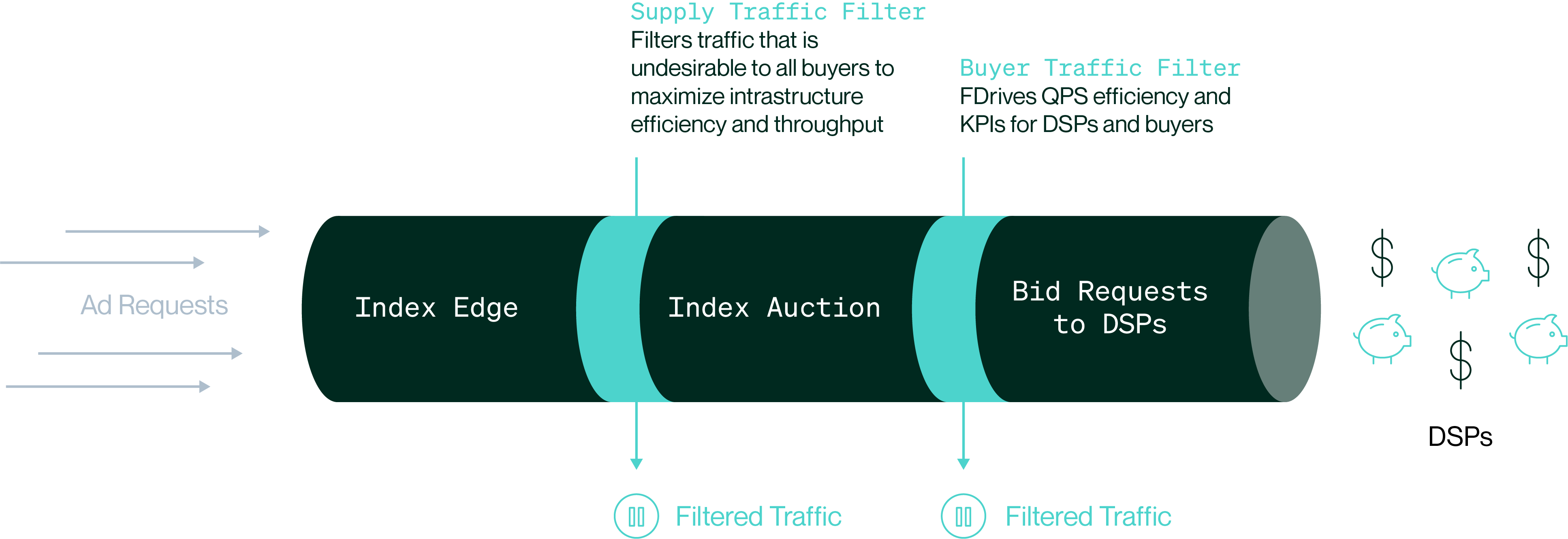
Our challenge was straightforward: what if we could predict which impression opportunities wouldn’t ever be likely to receive any bids, and effectively save the infrastructure that would be tasked with operating an unproductive auction? More importantly, could we accomplish this without any impact to media owners’ revenue?
This was the vision behind IX Traffic Filter, a machine-learning initiative that consists of separate but interlocking projects: Supply Traffic Filter and Buyer Traffic Filter.
Supply Traffic Filter
Supply Traffic Filter has two goals: ensure the exchange can scale to new heights, and make it more efficient. If we only run auctions that are likely to produce bids, we can maximize efficiency to media buyers at no cost to media owners, while protecting our infrastructure against traffic spikes.
Here’s how it works: we apply machine learning to historical auction datasets, building models that predict how buyers will bid on future ad requests. These models are deployed to every exchange node, and are used in real time to filter out requests that are anticipated to receive a “no-bid” from buyers. These models are accurate enough (and are constantly retrained!) to ensure that we can preserve revenue at rates >99%, while reducing exchange node load by double-digit percentages.
Learn more about machine learning and how our Supply Traffic Filter works.
Buyer Traffic Filter
While Supply Traffic Filter is focused on the exchange overall, the aim of Buyer Traffic Filter is to provide efficiency to media buyers: reduce their infrastructure costs, while sending them the most relevant traffic. Within each auction, we want to only send bid requests to buyers who actually want that traffic—without impacting their ability to buy (as in, we should not filter out requests that a buyer would have bid on). Buyer Traffic Filter sits further down the exchange than Seller Traffic Filter, and makes many filtration decisions per impression opportunity to make sure we route that auction to each buyer who wants to bid on it.
Buyer Traffic Filter works similarly to Supply Traffic Filter, but we use DSP-specific bidding data to make filtration decisions. The benefit to buyers depends on the flavor of DSP integration: QPS-uncapped DSPs will see efficiency gains, whereas QPS-constrained DSPs will see bid rates and fill rates increase as they will receive a more valuable traffic stream. This also affords them the opportunity to continue scaling as Index scales without having to make aggressive infrastructure investments at each stage of their growth.
What we’ve learned
So far, we’ve learned we can reduce our volume of auctions by double-digit percentages, with <1% impact to spend. We’ve learned we can pass through a small percentage of traffic to monitor our performance in real time, thus constantly retraining the system. And we’ve learned that this problem is the ideal candidate for machine learning techniques. We’re continuing to invest in IX Traffic Filter in order to be the most efficient exchange for buyers and the most resilient one for media owners. We’re grateful to be a safe haven for our customers, and we will continue to double-down in the face of uncertainty and push the envelope to keep the ecosystem strong.
Back to blog